Generative deep learning solves the aliasing problem of 3D imaging
In the context of the rapid development of informatization, digitization and intelligence, high-fidelity three-dimensional optical and visual imaging technologies play an increasingly important role in many industrial and consumer fields. Among them, the structured light three-dimensional imaging method represented by fringe projection can achieve accurate and rapid measurement of the three-dimensional shape and geometry of objects, and has received widespread attention and application in automated detection, robot perception, biomedicine and entertainment. Although this technology has high precision and high speed, it requires precise synchronization between imaging devices (projection modules and cameras), which limits the application of structured light technology in large-format, arrayed and collaborative three-dimensional perception scenes. Associate Professor Lv Lei of Henan University of Technology, Associate Researcher Su Zhilong of Shanghai University and the research team of the National University of Defense Technology established a generative deep learning method to solve the fringe aliasing problem in asynchronous imaging by analyzing the imaging aliasing problem caused by removing synchronization constraints, and proposed a generative asynchronous structured light three-dimensional imaging technology.
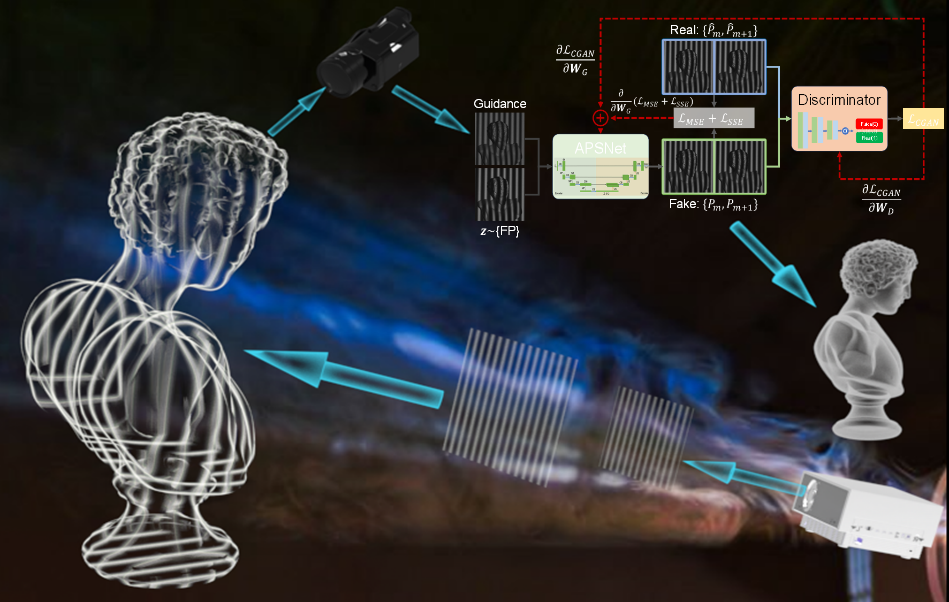
Traditional structured light technology relies on strict synchronization between the projection module and the imaging device to achieve accurate reconstruction of the three-dimensional scene. In asynchronous 3D imaging, each device is independent of each other, and the projection module can switch the projection pattern without being constrained by the exposure time of the camera device. This independence breaks the synchronization assumption in traditional methods, and the system can work more flexibly, reducing the synchronization requirements in practical applications, greatly expanding the application scope of structured light technology. However, it follows that multiple images will be captured during the camera exposure time, resulting in serious aliasing problems, resulting in accuracy loss of up to three orders of magnitude. Although the aliased imaging caused by this asynchronous has additive characteristics, it is affected by the coupling of multiple factors such as the camera exposure start time and the stripe switching time. These parameters are difficult to accurately predict or measure in practice, and have become a long-term problem of multi-source image aliasing that has restricted the development of asynchronous structured light technology.
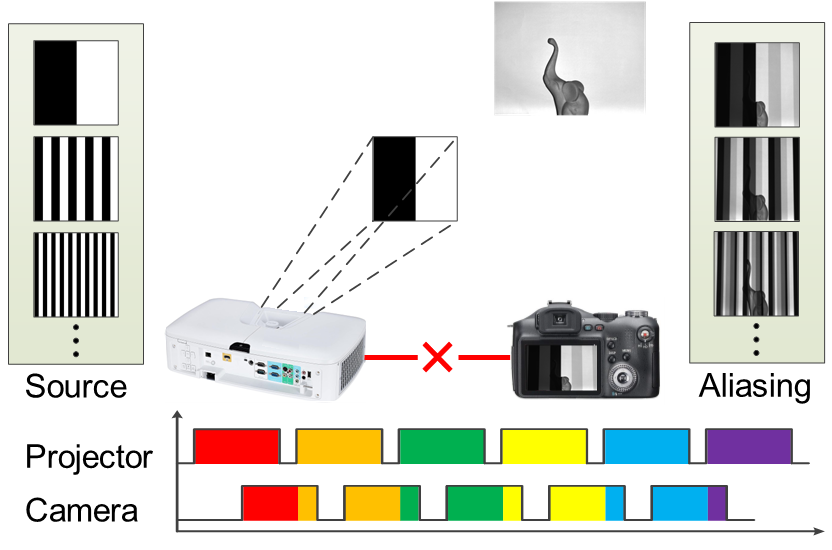
Under the topic of asynchronous imaging, stripe image aliasing is a long-standing challenge that has not been solved. The research team deeply analyzed the geometric and statistical characteristics of global additive image aliasing, and proposed to establish an effective generative deep learning method to solve this aliasing problem from the perspective of image generation under prior constraints. Specifically, taking the basic aliasing pattern as the starting point, the researchers found that the aliasing of asynchronous imaging has nothing to do with the spatial position of the pixel points, but is just the global accumulation of the light intensity of adjacent frames in an unknown combination. From the perspective of manifold geometry, the aliasing process does not deform the underlying structure of the image, so the aliasing separation process does not need to pay attention to the pixel position transformation problem. This actually provides a powerful and beautiful inductive bias-spatial equivariance prior, which naturally associates this problem with convolution. Therefore, a symmetric deep neural network model based on convolution can be designed to learn the intrinsic characteristics of stripes, which essentially guarantees the generalization ability of the model.
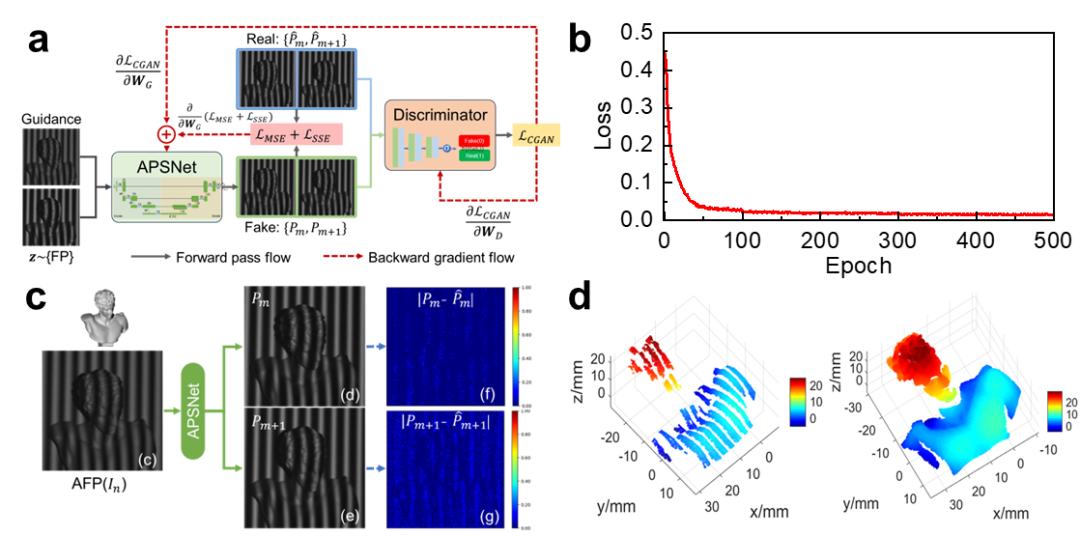
In order to use as little data as possible to build a model with strong generation ability, the research team introduced the generative adversarial learning framework (GAN) and further explored the statistical characteristics of stripe aliasing. On the basis of the adversarial learning framework, the stripe pattern prior is applied as a generation condition, and the underlying image similarity principle and the upper structural similarity principle are used to enable the model to efficiently learn non-aliased stripe information. Compared with general image generation models, the learning method based on the above strategy can effectively reduce the amount of data while ensuring the stability of the model training dynamics and strong generalization ability. By applying the classic phase shift method, the three-dimensional shape of the object can be accurately reconstructed from the generated stripe image. By using different objects and different stripe types for asynchronous three-dimensional imaging tests, it was found that the method proposed by the research team was comparable to the synchronous method in terms of accuracy. Compared with the asynchronous stripe reconstruction results, the accuracy was improved by up to three orders of magnitude.
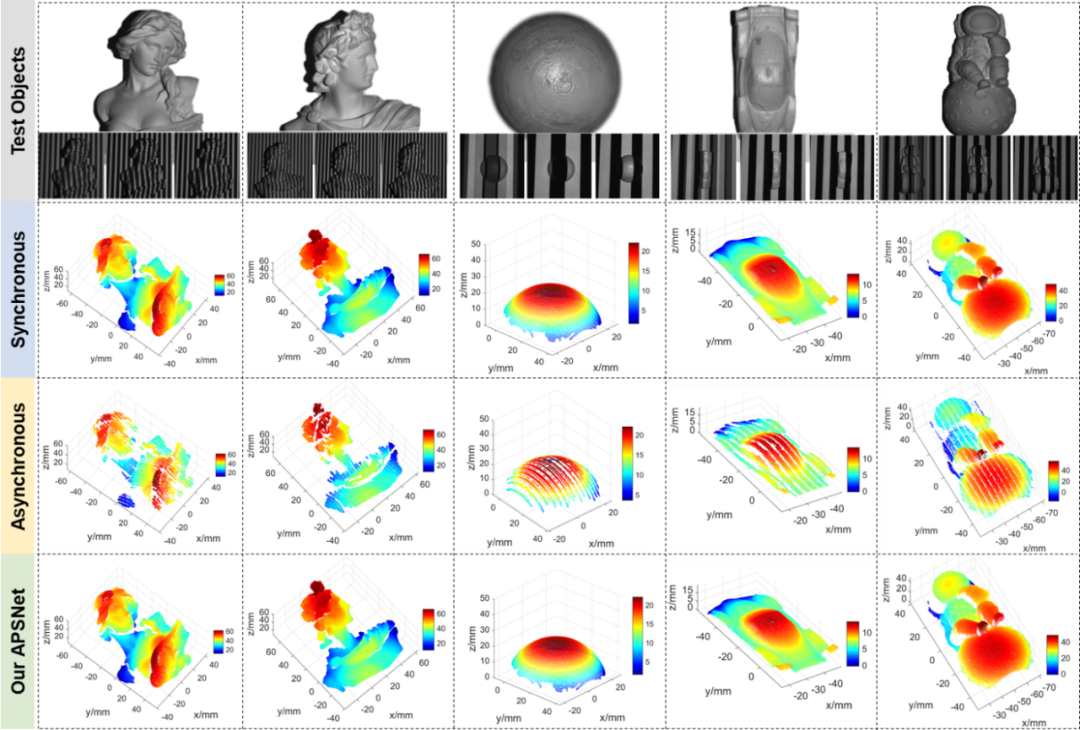
This achievement combines the concept of asynchronous imaging with generative deep learning methods, providing a new way to achieve highly flexible and scalable three-dimensional imaging perception in industrial and consumer scenarios. The proposed generative asynchronous three-dimensional imaging method can learn the intrinsic characteristics of structured light stripe images through a small amount of sample data under the guidance of “smart” priors, and can deal with asynchronous three-dimensional imaging problems in various scenarios with strong generalization. The new baseline framework provided can break the limitations of imaging devices and provide new ideas for the research and further development of structured light imaging.