photons are the new platform of artificial intelligence
Relying on powerful parallel computing network frameworks and GPU hardware support, artificial intelligence technology achieves high-speed computing and demonstrates superb performance in data processing, feature extraction, and nonlinear prediction. In recent years, artificial intelligence has gradually moved from science fiction to our daily lives, and many phenomenal products have emerged, such as AlphaGo, ChatGPT, and Sora. Artificial neural networks are the core foundation of artificial intelligence. Its design is inspired by our imitation of biological neural networks. The human brain is composed of tens of billions of neurons, which form a complex network through synaptic connections. Various information processing and task execution are achieved through input integration, threshold triggering, weight adjustment, and storage transmission. Similarly, artificial neural networks are based on electronic computing devices and simplify the imitation of biological neural networks from the perspective of information processing. Artificial neural networks are composed of many interconnected artificial neuron nodes and achieve preset goals through linear operations of weighted summation and nonlinear operations of activation.
In recent years, optical imaging technology has gradually entered a new era from traditional intensity imaging limited by the diffraction limit: computational imaging. Computational imaging can not only exceed the diffraction limit, but also obtain information such as phase, polarization, and spectrum [1]. Computational imaging can obtain physical information that cannot be obtained by traditional optical imaging by accurately modeling the physical process from the target to the sensor and using optimization algorithms to solve the inverse problem corresponding to the model. Computational imaging has broad application prospects in life sciences, astronomical observations, industrial detection, and other fields. The progress of artificial intelligence has greatly promoted the development of computational imaging. Deep learning has achieved high-quality results in establishing nonlinear forward models and solving high-dimensional ill-posed inverse problems through data-driven methods and has made significant progress in denoising, super-resolution, and low-light imaging. In addition, deep learning has shown amazing advantages in solving the common trade-off between quality and speed in computational imaging, which not only ensures the imaging effect but also compresses the speed to real-time levels. In 2023, the team of Academician Dai Qionghai of Tsinghua University proposed a self-supervised deep learning network DeepCAD-RT, which realized real-time three-dimensional microscopic denoising imaging of the mouse brain.
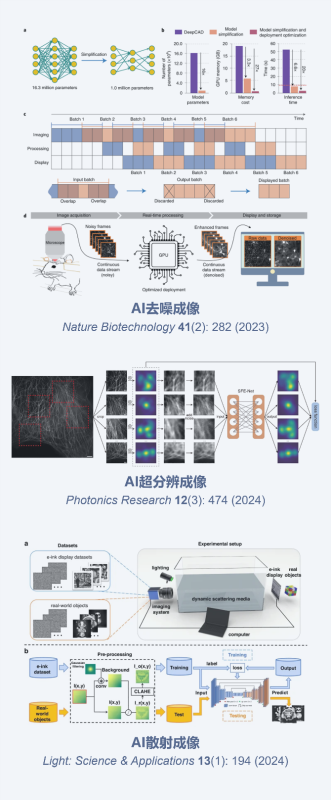
Another emerging direction is to use optical neural networks to achieve high-speed, high-dimensional, and high-precision imaging. The target light field information directly enters the optical neural network composed of multi-layer modulation devices in the form of light, eliminating the photoelectric conversion process, increasing the processing speed to the speed of light, and consuming very little energy, such as the denoising optical neural network framework proposed by the Ozcan team at the University of California, Los Angeles in 2024. In addition, optical neural networks can not only handle traditional computational imaging inverse problem tasks, such as scattering imaging, super-resolution imaging, and single-pixel imaging but also achieve some new tasks, such as erasing unexpected targets in imaging results. In addition, the collaboration of optical neural networks and digital neural networks can also achieve complementary advantages and solve complex problems such as long-distance transmission imaging.
To meet the needs of practical applications for the real-time generation of computer-generated holograms, MIT proposed to use supervised learning networks to accelerate hologram generation, and the trained network can achieve real-time generation of holograms on consumer-grade graphics processors. Deep learning has also made breakthrough progress in solving the speckle noise problem that has long plagued the development of computational holography. The unsupervised learning networks Holo-Encoder and 4K-DMDNet proposed by Tsinghua University have broken through the quality limitations of the modulated light field brought by the traditional CGH algorithm, and can automatically search for the encoding method of the CGH, realizing the high-speed generation of speckle-free high-fidelity light fields. In 2024, the Stanford University research team will combine deep learning-based CGH with metasurface gratings and dispersion compensation waveguides, making the landing of lightweight AR glasses possible. In addition, deep learning can also be used to optimize the compression process of CGH to meet the real-time transmission requirements of large amounts of data in three-dimensional scenes and help realize holographic communication as soon as possible.
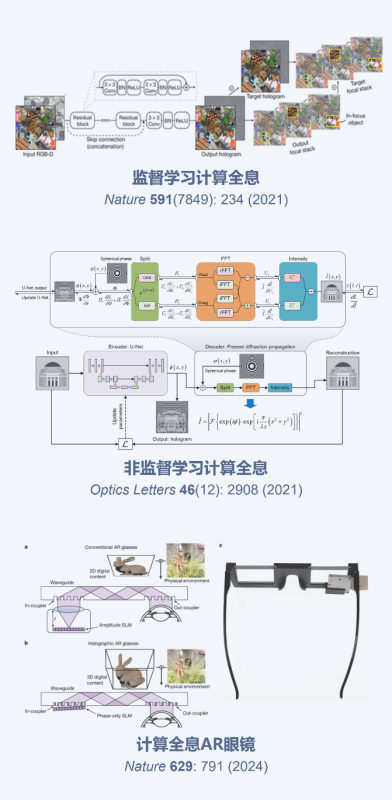
As an emerging interdisciplinary direction, although intelligent photonics has shown great potential and application prospects, it still faces many challenges in computing power, energy consumption, confidence, etc., and there is still a long way to go before large-scale commercial use. The development of intelligent photonics requires deep integration and innovation in materials, optics, computers, and other fields, as well as continuous attention and investment from industry, academia, and research. There is a long way to go, and we look forward to more scientific researchers devoting themselves to it and contributing more wisdom and strength to the progress of this cutting-edge field.