AI and lasers illuminate the way for manufacturing
Modern laser material processing systems generate a lot of data every day: on the one hand, these data can be used to monitor lasers and optical components; on the other hand, process monitoring also provides direct data from the material processing area. Today, this data is already used to monitor and record the quality of individual processing steps, helping users to evaluate changes in the processing process over time or across multiple machines.
1 The versatility of lasers
An important component of laser production system control is the cyber-physical system. In this system, computing, networking and physical production processes are integrated and fully automatically controlled through built-in feedback mechanisms. Additive manufacturing (3D printing) is a typical example, which usually uses lasers for physical processing, but the role of lasers is far more than that.
Since its development in the 1960s, lasers have provided exciting possibilities for the manufacturing industry. Lasers have a variety of applications and their characteristics are almost perfect. They can focus a lot of light energy on a small spot and generate a lot of heat on the target. Therefore, unlike many traditional mechanical and chemical industrial processes, laser processing is super efficient and does not require any additional chemicals.
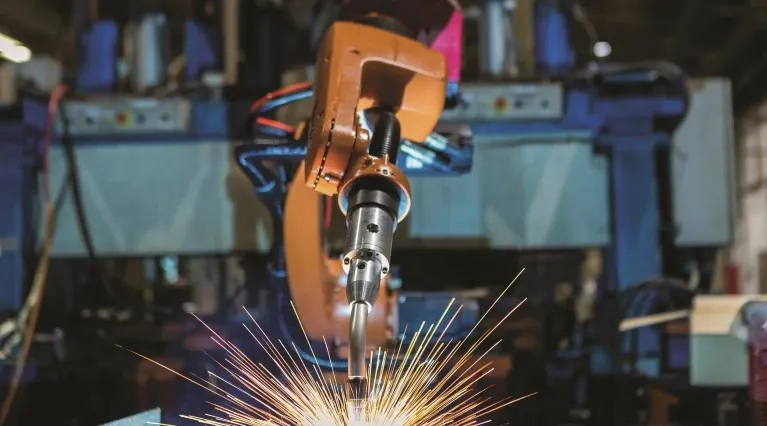
Lasers can generate pulses at extremely high rates, with each pulse lasting as little as a few tens of femtoseconds (10-15 seconds). This is faster than the rate at which energy is dissipated, so it is possible to microprocess materials smaller than a millimeter without causing thermal damage. As long as the laser wavelength is selected correctly, the laser can accurately process one of the materials while leaving the other intact. In addition, lasers can be used directly to weld components, cut metal sheets, or drill holes. Lasers can also be used more cleverly for surface polishing or texturing.
2 High-quality data
Because lasers are driven by electricity, they are easier to integrate into computer control systems and are ideal for cyber-physical systems. However, the range of characteristics of lasers that make such a wide range of applications possible also poses a major technical problem: how to find the best parameters for a specific job?
The traditional solution is trial and error based on the user’s intuition, but optimizing a laser system in this way can take months, which is simply not feasible at the scale required to achieve truly useful cyber-physical systems. To complicate matters further, even if the best configuration for a particular system to perform a specific task is determined, a configuration that works for one material may not work for another.
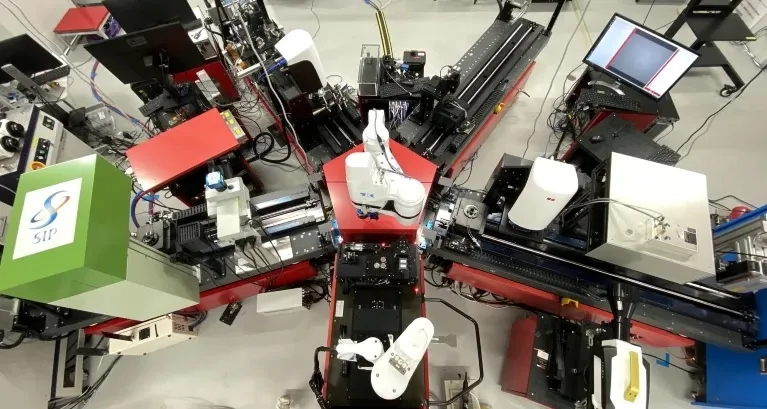
The researchers have applied their ideas to a number of laser production processes, including laser ablation: using short pulses of light to remove small amounts of material from a surface. In this case, they created high-quality datasets by firing light pulses of controlled duration at a solid target. This big data-driven approach has proven so fruitful that researchers at Kyushu University in Fukuoka, a project partner at the University of Tokyo, are already using it for semiconductor manufacturing.
3 Huge potential
Once the data has been collected, a deep learning algorithm can simulate the 3D topology created after multiple laser pulses at arbitrary positions and with arbitrary pulse energies. The team has already applied the system to a variety of materials, including dielectrics, semiconductors, and organic polymers. Currently, the Meister Data Generator system can autonomously search for various laser parameters using advanced algorithms such as Bayesian optimization, which can determine the next parameter to test while the experiment is running. Such high-quality data will have the potential to further transform laser manufacturing processes.
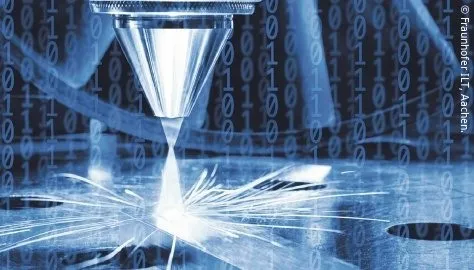
The next goal is self-learning of the machine. This is divided into four steps: First, the sensor generates data from the process. Then, the data is analyzed to make it easy to understand, that is, to interpret it based on the existing data. In the third step, the system simulates how the results of the process will develop. To do this, previous trends can be inferred, or the influence of certain parameters can be simulated. After that, the fourth step: system control will be enabled. So far, AI has been used mainly for quality monitoring and predictive maintenance of machines. Closed-loop control is the “next big thing”.
But with the increasing availability of digital process data, AI can do much more. Past data makes it possible to calculate new machining tasks and parameter ranges for specific materials in which the process should run well. When planning and setting up new machining processes, AI can save time and resources, and therefore money.